‘Rotating’ Memories Can Improve Machine Learning, Embry-Riddle Researchers Say
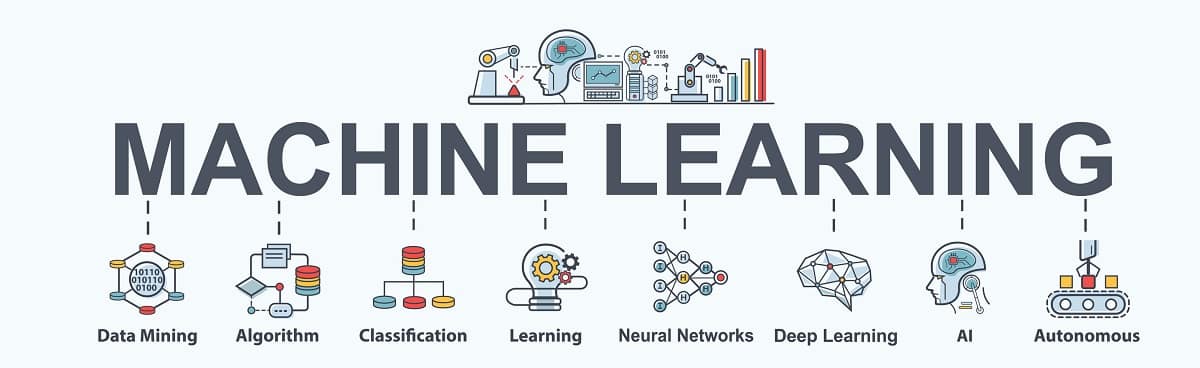
Advances in artificial intelligence, or AI, could someday help machines perform tasks typically assigned to humans such as weeding through thousands of aircraft identification signals to instantly detect rogue agents and improve aviation safety.
But first, machines must be able to master different concepts incrementally, or in stages, without forgetting one concept or the other – just as people can master the piano as well as the guitar. Understanding and implementing incremental learning in machines has been a major challenge in the field of artificial intelligence.
A new AI strategy proposed by researchers at Embry-Riddle Aeronautical University might improve incremental machine learning and avoid a common dilemma called catastrophic forgetting or catastrophic interference. The key to this advancement lies in rotating memories in an orthogonal (90-degree) direction – a process that researchers call memory orthogonality.
The strategy, published May 7, 2021 by the IEEE Internet of Things Journal, helps artificial neural networks (ANN) learn by presenting new memories that have been orthogonally separated from one another. Imagine a series of flash cards being held upright, then placed back down on a table. As each card is rotated back into place, the learner has a chance to process the new information before seeing the next card.
Interestingly, the Embry-Riddle work, based on mathematical studies of deep neural networks (DNN), seems to mimic biological processes, reported Houbing Herbert Song, ACM Distinguished Speaker, the director of the Security and Optimization for Networked Globe Laboratory (SONG Lab) and assistant professor of Electrical Engineering & Computer Science, and his Ph.D. candidate Yongxin Liu. In a recent study in mice published in Nature Neuroscience, Liu noted, Princeton University researchers found that new memories get rotated in an orthogonal fashion to protect them from incoming sensory inputs that might cause confusion.
“At least in mice, the biological brain seems to keep concepts separated into different directions so that it doesn’t get confused and start forgetting,” Liu explained. “We were excited to read about the Princeton study because we had previously discovered the same thing, as well as its mathematical essence, but in ANN, using a radically different approach.”
Liu hopes that his work will someday help minimize aviation cybersecurity risks.
Song envisions major potential for leveraging memory orthogonality to promote lifelong incremental AI learning. The approach could prove useful for tasks such as computer vision and natural language processing, for example. “This research will drive innovation in a range of AI application domains such as cybersecurity, autonomous systems and the Internet of Things (IoT),” Song said. “AI and neuroscience are driving each other forward, toward applications to benefit society.”
AI might help air traffic controllers distinguish between real versus counterfeit messages transmitted by legitimate aircraft or malicious cyber attackers, Liu said. However, current AI systems run into problems when they are confronted with too much incoming information. New input can overwrite existing knowledge, Liu explained, and then the AI model will begin to confuse everything that it has already learned. Yet, when two new pieces of information are orthogonal to each other, that separation seems to prevent confusion and enhances learning, he reported.
Song and Liu’s earlier AI work has included a strategy for detecting unauthorized unmanned aerial vehicles, or drones, and guiding them to land safely.